The ” IBM Quantum Hub at NTU,” established with funding support from the Ministry of Science and Technology (now the National Science and Technology Council) since January 2019, not only provides a service platform in quantum computing for the academic research and interdisciplinary exchanges in Taiwan, but also carries out a crucial mission of promoting quantum information science education in Taiwan.
“IBM Quantum Hub at NTU” held the first Quantum Computing Hackathon in 2020 at the Xitou Natural Education Area, which is under the NTU Experimental Forest Administration. The Hackathon activity was suspended in 2021 due to the outbreak of the coronavirus pandemic. The Quantum Computing Qiskit Hackathon Taiwan 2022 and the Quantum Computing Qiskit Hackathon Taiwan 2023 were both held on the 11th Floor of the Syntrend Building in Taipei. The 2024 NTU-IBM Quantum System Users Meeting, and the three-day Quantum Computing Qiskit Hackathon Taiwan 2024 were also held on the 11th Floor of the Syntrend Building from August 19 to August 21, 2024 (The event website is at https://quantum.ntu.edu.tw/?p=9048).
This year, the Hackathon event attracted more than 70 people to sign up upon registration opening, and most of the applicants already have experience using IBMQ, showing that the popularity and promotion of quantum computing has been effective in recent years. The competition committee selected 48 students to participate in the three-day competition. This event also invited three foreign universities to participate: Keio University in Japan, Yonsei University in South Korea, and Czech Technical University in Prague, the Czech Republic. Each foreign school selected 6~8 students to participate in the Qiskit Hackathon, making the event more international and diversified, as well as more challenging and watchable.
We invited Prof. Wen-Hao Chang, Program Executive Officer, NSTC Taiwan QuantumProgram Office, to give an opening remark and Dr. Kevin J. Sung, IBM Quantum, to give a keynote speech. To make the competition more challenging and interesting, the competition topics were announced to the contestants on site, and the competition team formation was completed on the spot. Participants were asked to compile their ideas into quantum programs using Qiskit, and present their results in English to other participants and the judges for evaluation. The winning teams were selected based on the criteria such as quantum technology complexity, problem originality, solution creativity, group cooperation and expression, future social contribution, etc.
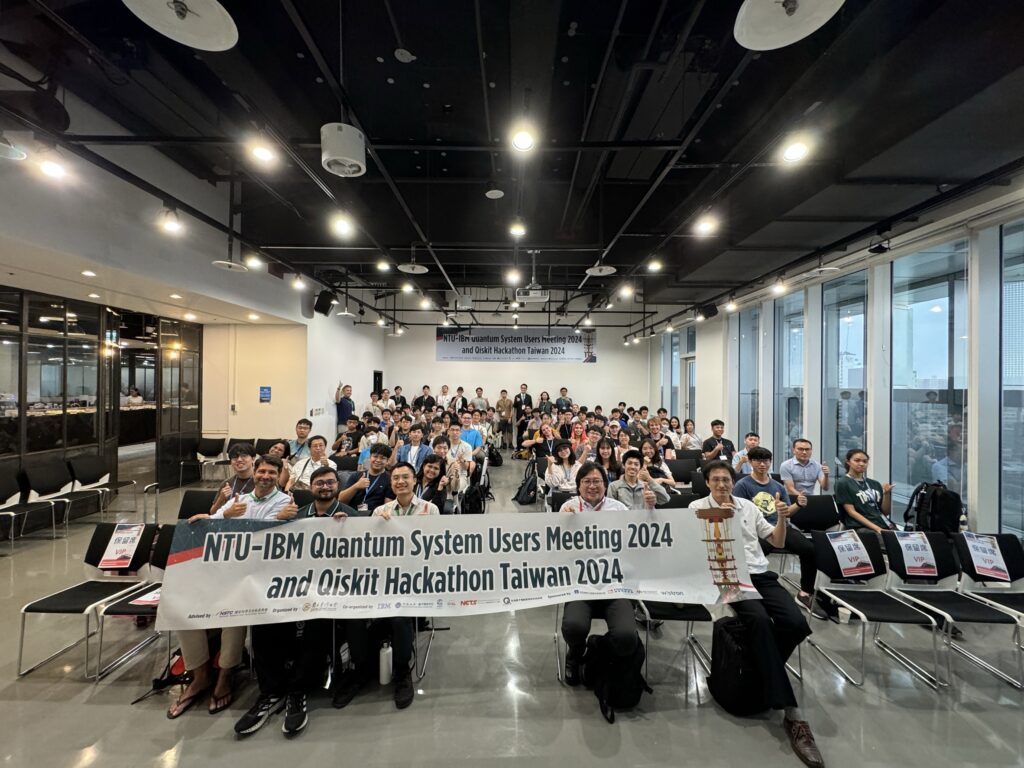
This event was mainly funded by the National Science and Technology Council and received assistance from IBM Quantum, which arranged coaches to guide competition students on-site and a quantum computing expert as a referee for competition presentations remotely. In addition, the co-organizers of the event included Chung Yuan Christian University Quantum Information Center, Center for Quantum Science and Engineering at NTU, Center for Advanced Computing and Imaging in Biomedicine at NTU, National Center for Theoretical Sciences – Physics Division, and Taiwan Association of Quantum Computation and Information Technology (TAQCIT). Domestic companies also actively sponsored and participated in the event. In addition to sending employees to participate in the Hackathon competition, they also provided generous prizes for the competition. In addition to Grand Jury Prize and NTU-IBM Quantum Hub Prize sponsored by the National Science and Technology Council, there are Enterprise Prizes from Foxconn, Formosa Plastics Corporation, Wistron, and Keysight Applied Technology. IBM Quantum also provided pencil pouches, note booklets, and Qiskit stickers to participants in the competition event. Cultivating experienced interdisciplinary talents and constructing and developing necessary applications are important tasks that Taiwan needs to focus on in the future development of quantum technology. Promoting the popularization of quantum computing education and learning is the key to further driving the country’s quantum technology industry to another higher level.
After three days of non-stop team efforts, the 2024 Quantum Computing Hackathon came to a wonderful conclusion. The fourteen participating teams brainstormed and discussed on topics such as optimization, quantum chemistry simulation, and quantum machine learning, and used Qiskit to implement their findings. The results of all the teams were comparably impressive, and in the end, 6 teams received the prizes.

Team 3 based their work on the Quantum-Train architecture, using quantum neural networks to train large classical neural networks. Quantum neural networks can represent classical neural networks with exponentially growing parameters using fewer qubits, significantly reducing the memory required for training. The team demonstrated superior results compared to classical training methods using the CIFAR-10 dataset, earning them the “Enterprise Special Prize.”

Team 4 won the “Enterprise Special Prize” with their project on “Hamiltonian Double Factorization.” They implemented double factorization of the Hamiltonians for chemical molecules such as H₂, N₂, and H₂O using the ffsim software package, calculating the ground-state energies. The students found that this method is faster than traditional approaches while maintaining good accuracy.

Team 12 won the “Enterprise Special Prize” with their project titled “Improved Quantum Image Encoding for Quantum Machine Learning with Information Mixer (Qix).” They based their approach on the Flexible Representation of Quantum Images (FROD), initially encoding quantum images into primary states, followed by using a quantum transformer, known as Mixer, for post-processing and classification tasks. The results demonstrated that a network architecture incorporating the Mixer mechanism (similar to FNet) has a stronger attention mechanism, significantly improving model accuracy and performance. Finally, a detailed comparative analysis showed that models utilizing the Mixer architecture performed notably better in image processing tasks than those without it.

Team 13 won the Enterprise Special Prize for their application of the HHL (Harrow-Hassidim-Lloyd) algorithm and its hybrid variant in solving linear systems of equations. The hybrid HHL method integrates classical and quantum computing to achieve shorter circuit depth and improve the precision of eigenvalue estimates. They implemented both algorithms for 2×2 matrices, demonstrating potential advantages over classical methods, particularly for larger matrices. In the future, this approach could be applied to numerical solving techniques and quantum machine learning.

Team 7 won the NTU-IBM Quantum Hub Prize with their project titled “AI Quantum Circuit Transpilation.” Quantum circuit transpilation is a crucial process in quantum computing, aimed at transforming abstract quantum circuits into a form that can be executed on real quantum hardware. This process involves optimizing circuit layout, routing qubits, and applying various transformations to enhance efficiency. The team focused on optimizing quantum circuits using custom transpilation techniques in Qiskit. They developed two main methods: a machine learning-based Pass Manager to collect three-qubit gates, and a custom Pass Manager specifically for the ZZFeatureMap circuit. The machine learning approach analyzes quantum circuits to identify recurring three-qubit gate operations and attempts to optimize them. Their results were compared with Qiskit’s default transpiler options (optimization levels 0-3) and the Qiskit AI Transpiler service, achieving a 5-10% reduction in both two-qubit gate count and circuit depth.

Team 1 won the “Grand Jury Prize” with their project titled “Large-Scale Quantum Circuit Compression.” Due to the error rates in current quantum hardware, quantum computing is limited to processing quantum circuits of fixed depth. To address this challenge, the team’s goal was to compress these circuits so they could run efficiently on existing quantum computers.
They applied the concept of quantum autoencoders to generate compressed quantum circuits on a simulator and successfully ran these circuits on real quantum computers. By simulating the evolution of hydrogen atoms, they verified their approach. After using the COBYLA optimizer with 200 iterations and applying a 15-layer ansatz, they managed to compress a quantum circuit with 3901 gates down to just 47 gates. Their method achieved over 98.1% fidelity in only 24.74 seconds for the largest circuit. When tested on IBM quantum computers, the mean squared error of the compressed circuit was below 0.025, compared to approximately 0.06 for the original circuit. Additionally, the original circuit took 5 seconds to run for the largest circuit, while the compressed version took only 2 seconds, saving more than half of the runtime.
